The buzz surrounding Big Data has been around for a couple of years now. But how does one analyze a massive amount of data to derive “good data” from it? It’s the good data that matters and processing it properly is a challenge. Although data analytics has existed for ages, it was never done in the large scale that we’re starting to see this year.
Big data analytics only became possible recently because of the following:
- Enhanced computing power
- Cost-effective storage
- Vast amount of distinct deep data sets
If you look at the employment pages, you will start noticing a lot of jobs listed for data scientists and data analysts. Big data R&D requires highly skilled individuals (and they are paid very well for this specialized skill). As a result, it’s pretty obvious that big data has gone from being a buzz word to big business.
Large enterprises no longer have a choice as big data is mandatory in order to stay relevant in the marketplace. No matter what industry you’re in, big data has the potential to open doors to new opportunities. But calculating the ROI of big data is complicated and difficult to accurately present to traditional business rationalists before they invest in analytics.
So what’s the compromise?
The only way to get everyone united for the cause is to get everyone to understand the potential of big data. In order to do that you have to first justify the investment before spending a significant amount of money on analytics.
The best way to justify the ROI is to start small and get results quickly.
Justify the Investment
The real value behind big data is identifying valuable information that was previously invisible. But assigning a value to data that hasn’t been discovered can be frustrating. The best way to move forward here is to make an initial investment to explore the data and look for potential opportunities.
If there is potential there, the second stage will be enhanced investment to develop a plan to extract more value from the data.
To get the returns that you require from big data, you have to first start asking the right questions. You should also be very specific. Once you have the question, develop suitable parameters to gather good data, develop methodologies for analytics, and evaluate findings.
There are two ways to assess the ROI for Big Data analytics:
- First of all you can compare the cost of running a big data project against other projects.
- Secondly, limit the scope of the big data and test the data by sampling it before making any commitments. If big data teams start out with a proof of concept, the project can be scaled down to be cost-effective.
Obstacles to measuring Big Data ROI:
- Defining the scope of big data exploration
- Forming a proper hypothesis
- Understanding how to process good data
- Finding skilled people to work in big data R&D
Big Data R&D – Strategy, Pilot, Production
Running a successful big data project starts with developing a concrete strategy. Data scientists need to get everyone on board to validate the business case. Once you’re ready to proceed, get IT and business professionals to unite under the same goal.
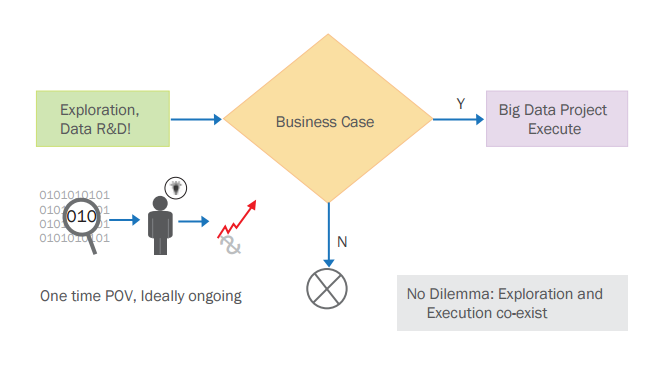
Source: impetus.com
Once the data analysts have done their job, the project will be ready for the pilot phase. In the pilot stage, the company will have to make decisions on the following:
- Suitable technologies
- Essential vendors
- Effective solution architecture
Running the pilot big data project will basically be your proof of concept. It will essentially demonstrate if you’re on the right track to executing a successful big data analytics project.
Once lessons are learned from the pilot stage, you will be ready to embark on a well-developed project. At this stage you are in the operating part of the process where you’ll be required to manage massive amounts of data coming in from various sources, design and apply big data clusters, and create and deploy new data analytics tools.
In the long run, the enterprises that will be most successful in big data analytics will be those that build efficient data R&D infrastructures and persevere through investigation, execution, tactics, and production.
Have you had success with big data analytics? How has big data impacted your sales?