AI is no longer just a promising tool—it’s a performance multiplier. Companies like Toyota, Siemens, and Microsoft are embedding AI into product design, simulation, and supply chain workflows—unlocking up to 60% gains in product performance and 50% improvements in market fit. The result? Faster development cycles, smarter decision-making, and hyper-personalized user experiences.
In 2025, the question isn’t “How do we add AI to this product?” It’s “How do our teams use AI to make better decisions together?” AI has become a core part of how high-performing product and engineering teams ideate, build, and evolve—shifting from feature delivery to intelligent system orchestration.
How Product and Engineering Have Evolved in the AI Era
In traditional software organizations, product managers and engineers operated with clear handoffs. PMs defined the what, engineering built the how. But as AI blurs the boundaries between problem definition and solution generation, this old model falls short.
As Microsoft highlights, modern product engineering demands tighter integration between teams and technologies. Engineers are no longer just coding features—they're curating intelligent systems. PMs aren’t just managing backlogs—they’re aligning models with business outcomes, data governance, and customer intent.
This transformation reflects a shift from feature delivery to system orchestration. AI-native teams make decisions together: when to automate, when to prototype, when to experiment, and when to scale. The result is not just faster shipping, but more strategic impact.
Strategic Shifts Driving AI-Native Product Development
According to McKinsey, five critical shifts define the modern AI-native product development lifecycle:
- Faster Time to Market: AI tools automate documentation, prototyping, and market research—reducing cycle times by up to 40%.
- Earlier Value Delivery: Feedback loops are integrated from day one through real-time sentiment analysis and user telemetry.
- More Ideas Validated: Automated A/B testing and simulation allow for more experiments and reduce HiPPO-driven prioritization.
- Expanded PM Roles: Product managers are becoming strategy owners, prototypers, and growth catalysts.
- Compliance and Quality by Design: Risk, ethics, and accessibility are embedded early through AI-assisted review tools.
These shifts don’t just create better products—they force organizations to rethink talent, incentives, and how value is defined.
What Sets AI-Native Teams Apart?
AI-native teams are distinguished by more than their toolsets. As DevOps.com notes, they are marked by product thinking, customer obsession, and systems fluency. Engineers are no longer execution agents—they are partners in strategy. They:
- Understand OKRs and business impact
- Participate in user research and feedback analysis
- Frame technical hypotheses as strategic bets
Similarly, PMs are now orchestrators of intelligent systems, not just feature owners. Their responsibilities include:
- Leveraging AI for decision augmentation, not replacement
- Interpreting data with nuance
- Embedding ethical guardrails into product design
This convergence elevates cross-functional excellence. When teams think and act together, speed becomes a byproduct of alignment.
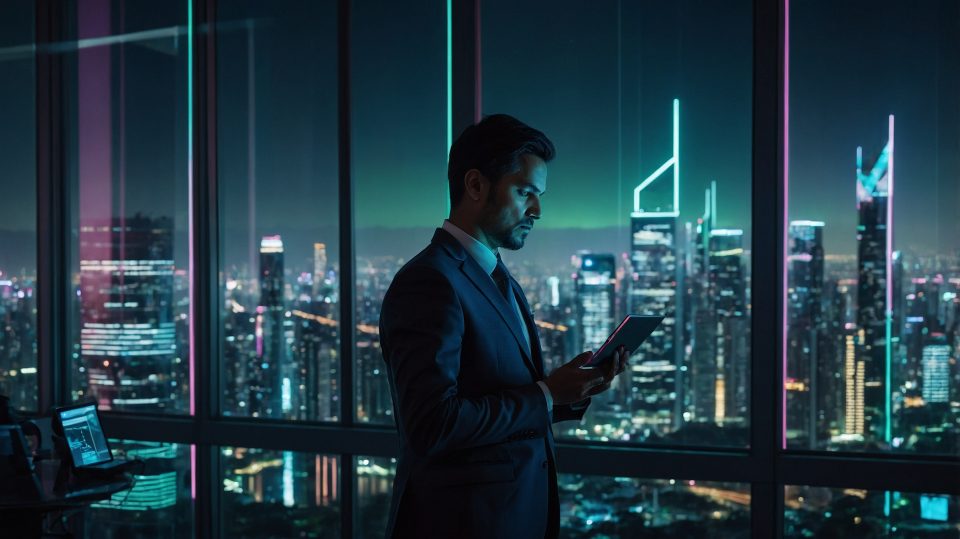
Organizational Impact and Industry Examples
What if the real differentiator for modern tech companies wasn’t just their AI capabilities, but how their product and engineering teams collaborate around them? In 2025, the conversation is shifting. AI is no longer a standalone tool or a late-stage integration—it’s a foundational element in how high-performing teams ideate, build, and evolve.
Top tech leaders are no longer asking, "How can we add AI to this product?" but rather, "How can our teams use AI to make better decisions, build smarter, and deliver value faster?" As outlined by McKinsey, companies that embed AI across the product development lifecycle are seeing faster time-to-market, deeper user insights, and stronger product-market fit.
Skills and Structures for the AI-Native Era
To scale these practices, organizations need to evolve both roles and mindsets. Here’s a breakdown based on role transformation insights from BloomVP's 2025 report on AI and product development , which explores how modern teams shift from traditional workflows to AI-enhanced collaboration.
Role | Traditional Focus | AI-Native Shift |
Product Manager | Requirements and roadmaps | Intelligent systems design and decision support |
Software Engineer | Clean code and delivery | AI orchestration and product insight |
Designer | User flows and interfaces | Prompt design and ethical UX |
QA / SDET | Manual and automated testing | Continuous, AI-augmented quality loops |
In parallel leaders should consider:
Encourage curiosity and hands-on AI experimentation
- Break down silos between product, design, engineering, and data
- Invest in upskilling for AI literacy across roles
Leading Through Collaboration and Intelligence
AI-native product teams aren’t just efficient. They’re intentional, ethical, and built for resilience. They know when to trust the model, and when to question it. They prioritize value over volume, outcomes over features.
At Intersog, we help companies design and scale these high-performance teams—blending product leadership, engineering expertise, and AI strategy to drive what’s next.
Because in 2025, the question isn’t whether your team is using AI. It’s whether they’re building with it—together.